Beñat Mencia
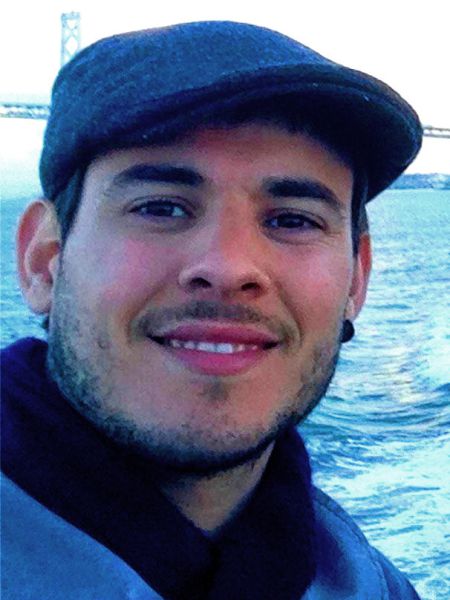
Beñat Mencia
PhD student in Prof Lamacraft's group
Office: 511 Mott Bld
Phone: +44(0)1223 3 37261
Email: bm485 @ cam.ac.uk
TCM Group, Cavendish Laboratory
19 JJ Thomson Avenue,
Cambridge, CB3 0HE UK.
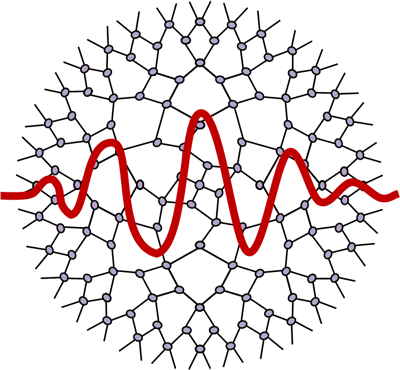
Research
My two main research lines are to do with learning of disentangled representations and quantum inspired data structures in machine learning.
Learning of disentangled representations
The key question is how to make machines represent information in a way that is interpretable to humans. Disentangled representations strive to reproduce the natural (by human intuition) characteristics of data in a mathematical space. Such representations are useful not only for standard downstream tasks such as supervised learning and reinforcement learning, but also for flexibly applying acquired knowledge across different tasks and learning from fewer examples. These are abilities humans have but machines struggle to achieve.
Quantum inspired machine learning
My second interest concerns the connection between physics and machine learning, in particular quantum inspired data structures. For example, audio data is wave-like and is sampled from a continuous signal. Although generative modelling of raw audio has made great strides lately, relational inductive biases relevant to these two characteristics are mostly absent from models explored to date. Quantum mechanics is a natural source of probabilistic models of wave behavior.
We built a generative model for raw audio that takes the form of a Stochastic Schrödinger equation describing the continuous time measurement of a quantum system, which is equivalent to the continuous Matrix Product State (cMPS) representation of quantum states in one dimensional systems. This representation is used to overcome the high dimensionality found in many problems in physics and it constitutes a deep Recurrent Neural Network architecture in which the systems state plays the role of a memory of past observations. This work is published in arxiv/1911.11879.
Physics
My most recent research in physics consisted of developing a new variational algorithm based on tensor networks implemented in TensorFlow, to seek the ground state of a quantum system, that should exceed the performance of state-of-the-art methods including DMRG.
I also have a track record studying vortex physics both in high-temperature superconductors and superfluids. In our last work we use numerical and analytical methods to study the ground state of an infinite system of vortex molecules in 2D.
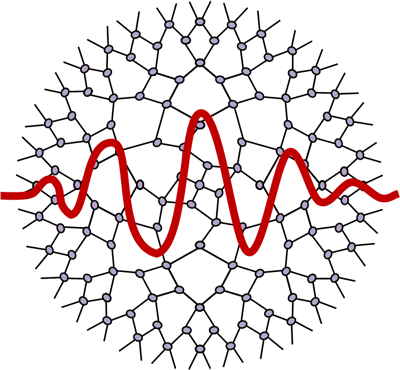
In Plain English
My first research interest learning of disentangled representations is to do with making machines represent information in a way that is similar to humans.
My second research line is to do with borrowing ideas from physics to solve problems in machine learning. More concretely, I am interested in using these ideas to develop a program that can generate new reallistic sound, by just learning from the sound it "hears".
My most recent research on physics consisted of developing new techniques to solve computational problems in a more efficient way.
My previous research is to do with swirls in some special fluids, called superfluids. If you take a glass of water and stir it with a spoon, you find that the water rotates creating a swirl in the middle. A superfluid is a fluid with some special properties. Among others, when you set it under rotation, it creates many swirls instead of just one. Interestingly, these swirls don't decay. Furthermore, under certain experimental conditions that can be tuned in the lab, these vortices appear to be pulled together in bound pairs forming "swirl-molecules". We study the behavior of these molecules.
Featured Publications
- SchrödingeRNN: Generative Modeling of Raw Audio as a Continuously Observed Quantum State
- Infinite Lattices of Vortex Molecules in Rabi-Coupled Condensates
- Electronic vortex structure of Fe-based superconductors: Application to LiFeAs
- Competing superconducting and magnetic order parameters and field-induced magnetism in electron-doped Ba(Fe1-xCox)2As2