Research Highlights
Artificial intelligence learns to distinguish between different topological band structures
The authors propose a machine-learning approach that can distinguish, without any form of previous knowledge, topologically distinct bandstructures.
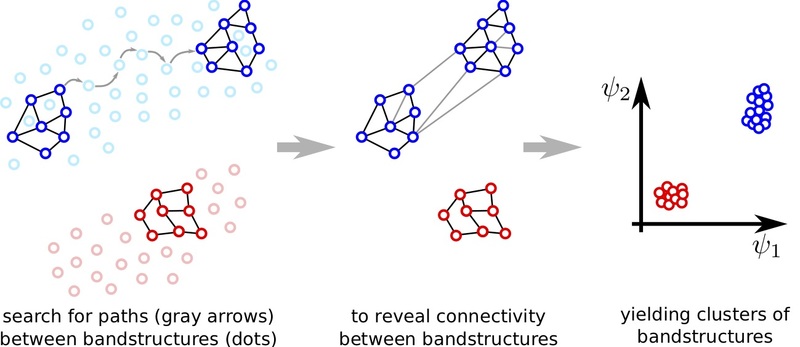
Instead of randomly sampling Hamiltonians of a symmetry class, light red/blue dots, a path finding algorithm is employed to retrieve adiabatic deformations (gray arrows) between Hamiltonians (dark red/blue dots). This constructs an effective graph, which is used to find an embedding that reveals the topologically distinct classes.
In modern many-body physics, the study of topological bandstructures, which is concerned with the question of whether two insulating phases can be smoothly ‘deformed’ into each other without closing the gap between bands, is one of the most active fields. This is motivated by the ongoing quest for a complete theoretical understanding of their classification, the rich physics these phases provide, and their potential applications, such as quantum-memory devices. Inspired by the success of artificial intelligence across fields, the authors construct a machine-learning setup that can determine whether two bandstructures are topologically equivalent. The key part is to use a path-finding algorithm, where paths refer to sequences of smooth deformations between bandstructures. The fact that it does not require any additional human input and that it is able to correctly predict the topological properties of several distinct paradigmatic models and types of topology is encouraging; it indicates that machine learning can be used to both expand our theoretical understanding of band topology as well as help uncover relevant material realizations of these exotic states of matter.
Non-Hermitian Boundary Modes and Topology M.S. Scheurer and R.-J. Slager, Phys. Rev. Lett. 124 226401 (2020)